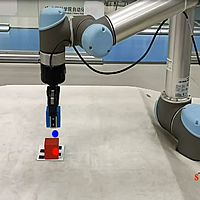
Manipulation skill learning on multi-step complex task based on explicit and implicit curriculum learning
Liu, Naijun; Lu, Tao; Cai, Yinghao; Wang, Rui; Wang, Shuo
Sci China Inf Sci, 2022, 65(1): 114201
Recently, remarkable progress of deep reinforcement learning (DRL) with regard to robot skill learning has been witnessed. However, manipulation skill learning on multistep complicated tasks with DRL still poses great challenges owing to its higher exploration of state-action space and uninformative sparse rewards. To alleviate these problems, some studies explored reward shaping to guide the learning process toward good solutions, which inevitably requires significant expert knowledge and manual efforts. Other study overcomes exploration with demonstrations which generally is costly and may result in suboptimal performance. Curriculum learning, which starts learning small domains with easier aspects of tasks and then gradually increases the difficulty level, is also applied to learn relatively easy manipulation skills. Another method called hindsight experience replay (HER) in hindsight imaged that every state that the agent finally reached was actually a goal, which showed appealing performance in some manipulation tasks with only sparse rewards available. However, it is still quite challenging for HER to directly train policies for multi-step manipulation tasks (e.g., picking and placing several objects).