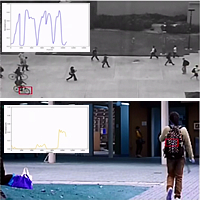
Reverse erasure guided spatio-temporal autoencoder with compact feature representation for video anomaly detection
Zhong Y H, Chen X, Jiang J Y, et al
Sci China Inf Sci, 2022, 65(9): 194101
Video anomaly detection aims to learn normal patterns and identify the samples deviating from normal patterns as anomalies. In early research, methods based on handcrafted low-level features have been widely studied. However, the representation power of low-level features is insufficient for describing various patterns, causing a bottleneck in handcrafted-feature-based anomaly detection. Recent methods are typically used to build reconstruction or prediction models based on deep learning to represent normal frames and detect anomalies based on the representation error. However, most existing detection methods based on deep learning adopt the loss function, such as l1-norm and l2-norm, to calculate the reconstruction or prediction error. In these methods, all pixels in the frame are processed equally, that is, the model loses its focus and does not prioritize learning and reconstructing the complex regions that are difficult to reconstruct during training. Consequently, the model may not be able to obtain reconstructed image with high quality foreground, since the simple background pixels dominate the optimization of model. Unfortunately, such issue may reduce the performance of anomaly detection, because the foreground is more important than the stationary background in anomaly detection. Further, existing reconstruction methods attempt to minimize the difference between the reconstructed frame and its ground truth. Although similarity is guaranteed in the pixel or even latent space, it is a one-to-one constraint, which ignores the similarity of different normal frames.